ML Governance is often discussed either in abstract terms without practical details or using detailed AI ethics examples. This talk will focus on the day-to-day realities of ML Governance.
How much documentation is appropriate? Should you have manual sign-offs? If so, when and who should perform them? When is an escalation needed? What should a governance board do? What if you are in a regulated industry? How can MLOps help? And most importantly, what is the point of all this governance and how much is too much?
This talk will show how each organisation can best answer these questions for their own context by referring to examples and public resources.
More about the speakers
As a Lead ML Engineer at Thoughtworks, Meissane advises and develops innovative Data Science and Machine Learning solutions from proof of concept to production. She has gained expertise setting up innovation frameworks and conducting fast cycle proof of concepts. Her primary area of expertise is in MLOps and Data Science.
Ryan Dawson is a technologist passionate about data. At Thoughtworks, he works with clients on large-scale data and AI initiatives, helping organizations get more value from data. His work includes strategies to productionize machine learning, organizing the way data is captured and shared, selecting the right data technologies and optimal team structures, as well as writing the code to make it happen. He has over 15 years of experience and, as well as many widely read articles about MLOps, software design, and delivery. Author of the Thoughtworks Guide to Evaluating MLOps Platforms.
About the speaker
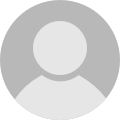
Meissane advises and develops data science and machine learning solutions from proof of concept to production. / Ryan works with clients on large-scale data and AI initiatives, helping organizations get more value from data.